According to
Gartner Peer Insights Reviews and Ratings, Microsoft (Power BI), Tableau, and Qlik are frequently the industry leaders in analytics and business intelligence platforms. However, how do these top platforms improve the field of data analytics, what distinguishes
them, and what are their alternatives?
Introduce yourself to the world of data storytelling. This powerful approach enables businesses, particularly those in the banking industry, to effectively and precisely communicate insights derived from their data. A new era of data presentation has emerged
thanks to visualization tools like Power BI, which enable powerful narratives that influence strategy and decision-making. In this article. let us explore the nuances of data storytelling and its significant applications in the banking industry, as demonstrated
by an engaging Power BI case study.
What is Data Storytelling?
Data storytelling is a form of conveying a message that combines data visualization and narrative techniques to make complex data stories that captivate and engage people. The audience can understand the essence of the data thanks to this synthesis, which
makes it understandable and relatable. Fundamentally, data storytelling aims to close the gap that exists between the tangible and the abstract, as well as between the quantitative and qualitative domains. Let us explore this in detail.
The Role of Data Visualization
Data visualization, which involves converting datasets into visual formats like charts, graphs, maps, and infographics, is central to data storytelling. These visual elements are essential in data storytelling because they make complex information easy to
understand. Data visualization reveals hidden patterns, trends, and relationships in the data, laying the groundwork for the narrative.
Exploratory vs. Explanatory Visualization
Data visualization is divided into two types: exploratory and explanatory. This split is critical in determining the approach to data storytelling:
-
Exploratory Visualization implies the technique which is used during the investigative phase of data analysis. When the narrative has yet to be discovered, exploratory visuals can help reveal the story hidden within the data. Questions such as "How does
sales revenue change over time?" or "Why did sales revenue spike in a particular season?" are addressed through this explorative process, setting the stage for the narrative to unfold.
-
Explanatory Visualization is used when the story is complete and the goal is to communicate specific insights to the audience. The visuals are designed to convey specific elements of the story or the entire narrative, ensuring that the audience understands
the message intended by the data storyteller.
See the diagram to understand the intertwining of these two with data storytelling:
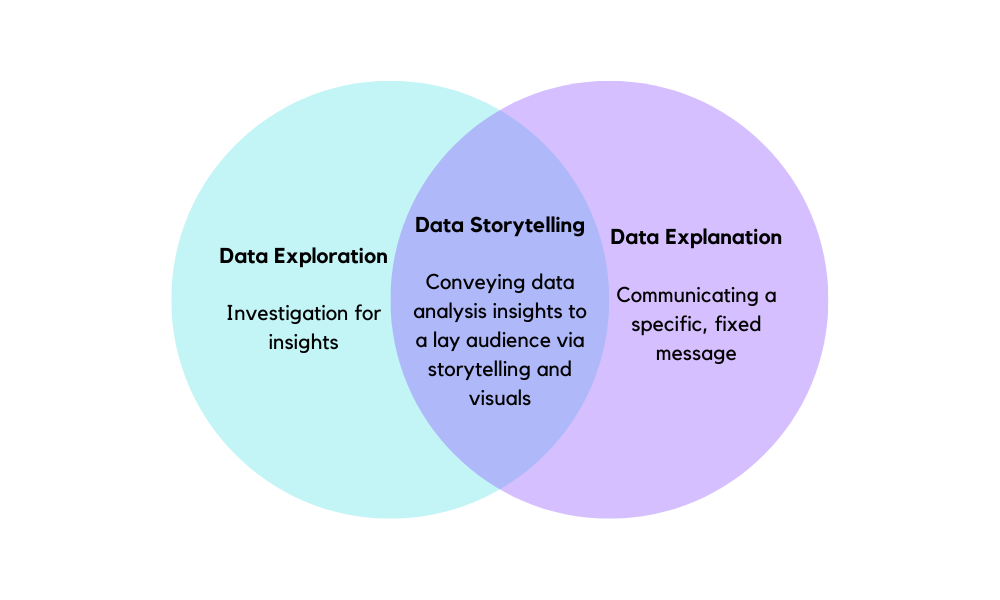
Narrative Structure Peculiarities
Effective data storytelling has a narrative structure that includes a clear beginning (introduction to the problem or context), middle (data analysis and insights), and conclusion (recommendations).
Unpacking the Components of Data Storytelling
-
Identifying the Audience: Customizing the narrative and visuals to reflect the audience's background and interests.
-
Defining the Story: Creating a coherent and concise narrative that addresses a specific business issue or goal.
-
Choosing the Right Visualizations: Choosing relevant types of charts, graphs, and visuals to highlight key insights and trends.
-
Adding Context: Providing context and explanations to help the audience understand the importance of the data.
-
Creating an Emotional Connection: Incorporating storytelling elements that evoke emotion, making the data more relatable and memorable.
A Power BI Case Study in the Banking Sector
Let’s consider a particular example of how Data Storytelling works in the area of banking.
Customer retention in this sector is equally significant as customer acquisition. A major bank was in a situation where the churn rate was on the rise, threatening to take away its market position and revenue. To meet this challenge, Power BI came in, which
is an interactive tool that offers high-end data analysis and visualisation functionalities. This case study highlights the strategic application of Power BI by the bank to enhance customer retention by pinpointing patterns in transactional data with strong
predictive power concerning potential churn.
Problem Statement
So, assume the bank realized a worrying pattern: over time, the number of customers who departed for rivals was rising. The churn rate not only showed how much it negatively affected the bank’s profitability but also highlighted possible shortcomings in
customer satisfaction and engagement. The difficulty was to sort out huge volumes of transactional data along with customer demographics and behaviour to detect the root causes of churn. Consequently, the objective of a data-driven strategy should not only
have been retention but increased satisfaction overall.
Data Analysis
Right on the spot, the bank started a journey through the world of advanced analytics with Power BI. To begin with, we integrated transactional data, customer demographics, and behavioural patterns to form a single dataset. The advanced analytics features
available in Power BI enabled them to deeply explore this dataset and bring out crucial churn trends and patterns. Among them, they could track down the customer segments that are more likely to leave, types of transactions indicating future churn, as well
as periods with heightened churn activity.
Visualization
After analyzing the data, the next step is to make those insights usable. Power BI's interactive dashboards come into play, providing clear, dynamic data visualizations. These dashboards highlight customer segments that are at higher risk of churn and reveal
the factors that influence a customer's decision to leave. The bank makes data visually appealing and enables these insights to be disseminated across departments to ensure decision-makers have the information they need to take decisive action.
Narrative
Nevertheless, data alone, no matter how accurately analyzed, cannot inspire action without a compelling narrative. The bank made a story around the data, emphasizing the critical importance of customer retention, the tangible impact of churn on the bank's
revenue, and the strategies identified to deal with this churn. This narrative served to inform and motivate the entire organization towards a unified goal.
Outcome
Strategically applying insights gained from Power BI transformed the bank's approach to customer engagement. With detailed analytics and clear visualizations, the bank launched targeted marketing campaigns and personalized offers based on the needs and behaviour
of high-risk customer segments. As a result, customer churn rates dropped significantly, while customer satisfaction and loyalty increased.
In the process, the bank addressed the pressing challenge of reducing customer churn and reaffirmed its commitment to leveraging technology for strategic advantage. This case study demonstrates how Power BI can be a powerful tool for understanding and increasing
customer loyalty, ultimately achieving long-term success in the highly competitive banking industry.
Main Inferences
Our case looks at centres on a major bank that leveraged Power BI to tackle rising customer churn quotes, aiming for strategic improvements in customer retention and delight. The bank set ambitious objectives for its Power BI implementation:
Data Extraction Speed: Aiming for a 50% discount in extraction time, from 60 minutes to 30 minutes, improving performance.
ETL Process Efficiency: Achieving a 96% fulfilment price for ETL jobs, with a 30% lower in information transformation time, ensuring reliability and velocity.
Data Model Optimization: Setting a refresh time of 15 minutes for statistics models and a query reaction time of underneath 3 seconds, optimizing performance.
Report Rendering Speed: Targeting a document load time of underneath 6 seconds and visualization rendering of under 2 seconds, enhancing UX.
Data Compression Ratio: Aiming for as a minimum a 10:1 information compression ratio, optimizing storage usage and lowering costs.
Data Governance Compliance: Maintaining a data lineage documentation score of a minimum of 92% and data access management effectiveness rating of 98%, ensuring protection and compliance.
Conclusion
One of the most effective tools for businesses to communicate insights and drive action is data storytelling. There are many visualization platforms like Power BI, and Tableau, among others, that have built-in powerful visualization capabilities that allow
users to create compelling narratives. Hence, the craft of data storytelling, complemented by influential visual presentation approaches, is an essential factor for any organization that aims to unlock its true data value.